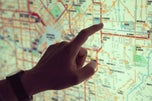
Waste management is a vital public service ensuring environmental health and urban cleanliness. However, the industry faces significant challenges, including rising fuel costs, inefficient collection routes, and growing demands for sustainability. Traditional methods of route planning often result in increased operational expenses and greenhouse gas emissions, exacerbating environmental concerns.
Innovative technologies such as AI, GIS, and automation offer new possibilities for route optimization, enabling waste management companies to plan efficient collection routes, improve resource allocation, and reduce environmental footprints. This paper investigates how route optimization methods can address these challenges, highlighting successful applications and future opportunities.
Challenges in Waste Management Routing
Inefficient Route Planning
Traditional route planning relies on static schedules and limited data, often leading to unnecessary mileage and time inefficiencies.
Rising Operational Costs
Fuel costs, vehicle maintenance, and labor expenses represent substantial financial burdens for waste management companies.
Environmental Impact
Inefficient routing contributes to excessive fuel consumption and greenhouse gas emissions, undermining sustainability goals.
Service Demand Variability
Fluctuating waste generation patterns and growing urban populations create dynamic service demands that traditional methods struggle to accommodate.
Route Optimization Techniques
- Geographic Information Systems (GIS)
GIS technology integrates spatial data with route planning, enabling waste management companies to create efficient and data-driven collection routes.
- Capabilities:
- Map-based visualization of service areas.
- Identification of optimal collection points.
- Real-time route adjustments.
- Example: GIS tools calculate shortest paths, considering road conditions and traffic patterns, to minimize travel distance.
- Artificial Intelligence and Machine Learning
AI algorithms analyze historical and real-time data to optimize routes dynamically. Machine learning enables continuous improvement in routing efficiency.
- Applications:
- Predictive modeling of waste generation patterns.
- Adaptive routing based on traffic and weather conditions.
- Clustering of collection points to reduce redundancies.
- Example: AI-powered systems reconfigure routes in response to real-time changes, such as road closures or fluctuating waste volumes.
- Vehicle Routing Problem (VRP) Algorithms
VRP algorithms solve complex routing challenges by determining the most efficient way to service multiple locations.
- Techniques:
- Exact Algorithms: Optimal solutions for small-scale problems.
- Heuristic Methods: Approximate solutions for larger, more complex scenarios.
- Metaheuristics: Advanced techniques such as Genetic Algorithms and Simulated Annealing for high-efficiency routing.
- Internet of Things (IoT) Integration
IoT-enabled smart bins equipped with sensors provide real-time data on waste levels, facilitating demand-based routing.
- Capabilities:
- Monitoring bin fill levels.
- Triggering collection only when bins reach capacity.
- Enhancing resource allocation.
- Example: IoT systems prioritize routes to areas with overflowing bins, avoiding unnecessary trips.
- Automated Fleet Management Systems
Automation streamlines fleet operations, ensuring optimal use of vehicles and resources.
- Features:
- Real-time vehicle tracking.
- Automated dispatching and scheduling.
- Maintenance alerts based on usage patterns.
Benefits of Route Optimization
Cost Efficiency
- Fuel Savings: Reduced travel distances lower fuel consumption.
- Labor Optimization: Efficient routes minimize overtime and idle time.
Environmental Sustainability
- Emission Reduction: Optimized routing decreases greenhouse gas emissions.
- Resource Conservation: Demand-based collection avoids unnecessary use of vehicles and equipment.
Enhanced Service Quality
- Timely Collection: Optimized schedules ensure timely waste pickup.
- Customer Satisfaction: Reduced missed collections and quicker response times improve user experiences.
Scalability
- Urban Expansion: Advanced routing systems adapt to growing service areas.
- Dynamic Demand: Flexible algorithms handle fluctuating waste volumes.
Case Studies
Case Study 1: Smart Waste Management in Barcelona
Barcelona implemented IoT-enabled smart bins and AI-powered routing systems to optimize waste collection. The initiative resulted in a 20% reduction in operational costs and a 30% decrease in emissions.
Case Study 2: Predictive Routing in Singapore
Singapore’s waste management authority adopted predictive analytics to anticipate waste generation patterns. The system improved route efficiency by 25% and reduced vehicle wear and tear.
Case Study 3: GIS-Driven Optimization in New York City
New York City integrated GIS technology into its waste collection operations, achieving significant fuel savings and improved service reliability.
Challenges in Implementing Optimization Methods
High Initial Costs
Adopting advanced technologies requires significant upfront investment.
- Solution: Explore public-private partnerships and government grants to fund technology adoption.
Data Integration
Integrating data from diverse sources can be complex and time-consuming.
- Solution: Develop standardized data protocols and interoperable systems.
Workforce Training
Employees may require training to operate new systems effectively.
- Solution: Implement comprehensive training programs and promote a culture of innovation.
Cybersecurity Risks
Connected systems are vulnerable to cyberattacks.
- Solution: Employ robust security measures and regular system audits.
Future Trends in Route Optimization
AI-Powered Autonomous Vehicles
Autonomous waste collection vehicles equipped with AI will enable fully automated and efficient routing.
Advanced Predictive Analytics
Enhanced AI models will predict long-term waste trends, supporting strategic planning and resource allocation.
Integration with Smart City Infrastructure
Waste management systems will integrate with broader smart city initiatives, enabling seamless coordination across urban services.
Conclusion
Route optimization methods, powered by AI, GIS, IoT, and automation, represent a transformative opportunity for the waste management industry. By addressing inefficiencies, reducing costs, and minimizing environmental impact, these technologies ensure sustainable and scalable waste management solutions. As urbanization and environmental challenges grow, investing in advanced route optimization will be essential for creating resilient and efficient waste collection systems.
References
- World Bank. (2020). Reducing Waste in Urban Systems. World Bank Publications.
- International Solid Waste Association. (2021). Smart Waste Management Strategies. ISWA.
- Deloitte Insights. (2021). The Role of AI in Urban Sustainability. Deloitte.
- McKinsey & Company. (2022). Optimizing Urban Waste Systems. McKinsey Insights.
- PwC. (2021). Automation in Waste Management: Trends and Benefits. PwC Insights.
- Siemens AG. (2022). Innovations in Waste Collection Technology. Siemens Publications.
- Frost & Sullivan. (2021). The Future of IoT in Waste Management. Frost & Sullivan Reports.
- IBM Corporation. (2021). AI Applications in Environmental Management. IBM Insights.
- Schneider Electric. (2021). Efficient Waste Collection Using IoT. Schneider Reports.
- MIT Technology Review. (2022). AI in Waste Management: Case Studies. MIT Press.
- European Commission. (2022). Sustainable Waste Systems in Europe. EC Publications.
- World Economic Forum. (2021). Smart Cities and Waste Optimization. WEF.
- Automotive News. (2022). Autonomous Vehicles in Waste Collection. Automotive News Insights.
- National Waste & Recycling Association. (2021). Trends in Route Optimization. NWRA.
- Accenture. (2021). Digital Transformation in Waste Management. Accenture Reports.