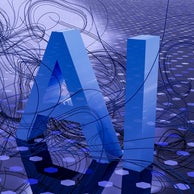
Navigating Security and Governance in SMB AI Adoption
Navigating Security and Governance in
SMB AI Adoption: Safeguarding Data and
Applications
Authored by Dr. Nicholas J. Pirro
Pyrrhic Press Publishing | www.pyrrhicpress.org
February 18, 2025
Abstract
As small and medium-sized businesses (SMBs) explore AI adoption, security and governance
concerns often present significant barriers. Data protection, unauthorized usage, and compliance
issues can undermine confidence in AI tools. This paper examines the critical role of security and
governance frameworks in AI deployment for SMBs, offering insights into successful practices that
safeguard data integrity and ensure responsible AI application development.
Understanding the Security Concerns
For SMBs, the prospect of integrating AI often triggers concerns about data leaks, unauthorized
access, and potential breaches (Westerman et al., 2014). Unlike large enterprises with dedicated
cybersecurity divisions, SMBs may lack robust infrastructure to monitor and protect AI-driven
processes. This creates hesitation, limiting AI experimentation and adoption (Pyrrhic Press, 2024).
Key security concerns among SMBs include:
• Data Privacy: Sensitive customer data processed by AI models may be exposed to external
systems during cloud-based operations (Smith, 2023).
• Unauthorized Model Access: Without proper access controls, employees may use AI tools
inappropriately, risking data leaks or inaccurate outputs (Anand, 2025).
• Model Hallucinations and Fabricated Data: AI models can occasionally produce false
information or cite non-existent sources, raising legal and reputational risks (Pyrrhic Press,
2024).
Governance Challenges in SMBs
Governance structures define how AI tools are implemented, monitored, and updated. However,
SMBs frequently lack formal processes to oversee AI applications. This can result in fragmented
deployments, redundant tools, and poorly integrated solutions (Brynjolfsson & McAfee, 2017).
Without governance, employees may develop isolated AI-powered applications—useful in the short
term but disruptive to long-term data consistency and security.
Common governance gaps include:
• Lack of Standardized Access: Employees deploying AI tools independently, often
bypassing IT oversight.
• No Audit Trail: Difficulty tracking AI usage and outputs, complicating compliance checks.
• Inconsistent Application Development: Non-technical staff creating AI-powered
applications without aligning with enterprise standards (Pyrrhic Press, 2024).
Best Practices for SMB AI Security and Governance
1. Access Control Frameworks:
Implementing tiered access ensures that only authorized employees can interact with AI tools. For
instance, assigning different permissions based on job roles can reduce the risk of sensitive data
exposure (Anand, 2025).
2. Centralized AI Platforms:
Platforms that consolidate AI tool usage under a single system improve oversight and auditability.
SMBs can benefit from portals that log AI interactions, enabling IT teams to review usage patterns
(Pyrrhic Press, 2024).
3. Data Handling Policies:
SMBs should enforce policies requiring anonymization of sensitive data before AI processing.
Ensuring AI models operate within secure environments, such as private cloud systems, can further
mitigate risks (Westerman et al., 2014).
4. Human Validation of Outputs:
While AI can accelerate research and content creation, human review remains vital. Employees
should validate AI-generated outputs, particularly in customer communications or legal
documentation (Smith, 2023).
5. Regular Security Audits:
Periodic audits help detect vulnerabilities in AI systems, ensuring adherence to internal data
protection policies and regulatory requirements (Brynjolfsson & McAfee, 2017).
Case Study Insights
A regional accounting firm adopted AI-powered financial analysis tools but initially faced resistance
due to data privacy fears. By deploying a centralized AI platform with role-based access, the firm
achieved secure usage while enabling employees to automate report generation. This resulted in a
35% reduction in administrative hours without compromising data integrity (Pyrrhic Press, 2024).
Similarly, a logistics company introduced an AI chatbot for customer inquiries but mandated
human review for sensitive responses. This hybrid approach safeguarded the company against
inaccuracies while streamlining customer support processes (Smith, 2023).
Conclusion
Security and governance serve as the foundation for sustainable AI adoption within SMBs. By
implementing robust access controls, adopting centralized platforms, and fostering a culture of
human-AI collaboration, SMBs can harness AI's potential while safeguarding their operations. As AI
models evolve, SMBs that proactively address security and governance will position themselves for
resilient, long-term success.
References
Anand, R. (2025). Internal AI adoption and workforce transformation at Strive Corporation. Internal
Research Report.
Brynjolfsson, E., & McAfee, A. (2017). Machine, platform, crowd: Harnessing our digital future. W.
W. Norton & Company.
Pyrrhic Press. (2024). Business leadership case studies: Real-world applications of AI/ML in small
enterprises. Pyrrhic Press Publishing.
Smith, J. (2023). Unlocking the AI frontier for small businesses. Journal of Business Technology,
45(2), 34-48.
Westerman, G., Bonnet, D., & McAfee, A. (2014). Leading digital: Turning technology into business
transformation. Harvard Business Review Press.