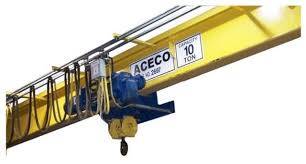
In industries that rely on heavy machinery, such as crane systems, equipment downtime can lead to significant productivity losses and increased costs. Predictive maintenance, which focuses on preventing unexpected failures through data-driven insights, has emerged as a solution to these challenges. Predictive maintenance systems analyze operational statistics (such as load, uptime, and machine status) and correlate them with past outage data to identify patterns that signal impending failures (Lee et al., 2020). This paper explores the need to integrate multiple data sets—specifically outage records and operational statistics—to generate a timeline that predicts when and why crane systems experience downtime.
Background
Cranes are vital for industrial activities, ranging from manufacturing to logistics, and the consequences of downtime are costly. Traditionally, maintenance strategies have been reactive—performed only after a breakdown has occurred. However, this reactive approach often results in unplanned downtime and higher maintenance costs (Mobley, 2002). Predictive maintenance, in contrast, uses data analytics to predict failures and schedule timely maintenance before failures occur (Jardine et al., 2006). A critical aspect of predictive maintenance is the ability to consolidate different data sets—such as outage data, operational load, and sensor readings—into a comprehensive system for detecting patterns and anomalies (Ahmad & Kamaruddin, 2012).
Methodology
Data Collection and Integration
To implement a predictive maintenance strategy, we need to integrate two primary data sets: outage data and operational statistics. Outage data provides historical information on when crane systems experienced downtime, whether it was planned or unplanned. Operational statistics, on the other hand, include various machine metrics, such as uptime, load, and sensor readings, which provide insight into how the crane was functioning prior to an outage (Grall et al., 2002). Combining these two data sets allows for a clearer understanding of the conditions leading to system failures.
Outage Data
Outage data is critical for identifying patterns in system failures. For this analysis, we differentiate between planned and unplanned outages. Planned outages are scheduled and typically occur during routine maintenance checks. Unplanned outages, however, are unexpected and result from equipment failure. Both types of outages need to be tracked, including the date, time, duration, and root cause (Mobley, 2002). These outages are then sorted chronologically to construct a timeline, allowing us to track when downtime occurred.
Operational Statistics
Operational statistics refer to the data that reflects how the crane was functioning at the time leading up to the outage. Key factors include load (e.g., weight being lifted), uptime (e.g., how long the crane has been operating continuously), and sensor readings (e.g., from cameras, radar, or photoelectric sensors). By correlating these statistics with the outage timeline, we can identify significant trends. For example, we might observe that the crane tends to fail after four consecutive hours of operation under a heavy load. This operational data should be captured at regular intervals (e.g., every hour) to build a robust dataset for analysis (Ahmad & Kamaruddin, 2012).
Predictive Model Development
Once both the outage and operational statistics data sets are collected and integrated, we can begin to develop a predictive model. This model aims to forecast the likelihood of future outages based on the conditions observed prior to past failures (Jardine et al., 2006). The model uses historical patterns—such as frequent downtime after prolonged operation or excessive load pressure—to generate alerts when similar conditions arise. For instance, if the system detects that a crane has been running for four hours continuously with a heavy load, it may predict a potential failure and trigger preemptive maintenance actions.
Discussion
Integrating these data sets is the most important step toward building an effective predictive maintenance strategy. Merely collecting outage data without operational statistics may not provide a complete picture of why the failures occur. Conversely, operational statistics without outage records lack the necessary context to pinpoint failure causes. By combining these data sets, we can derive insights into failure patterns and develop timelines showing the sequence of events leading to downtime (Lee et al., 2020).
Moreover, tracking the cost of maintenance is equally important. Unplanned maintenance is generally more expensive than planned maintenance due to the urgency of repairs and the higher risk of further damage (Mobley, 2002). This cost information can also be incorporated into the predictive model, helping organizations not only anticipate equipment failures but also manage the financial impact of maintenance activities.
Conclusion
Predictive maintenance offers an effective way to reduce downtime and maintenance costs in crane systems by using historical data to anticipate equipment failures. By integrating outage data and operational statistics, organizations can develop robust predictive models that trigger maintenance before failures occur. The next step is to continue refining these models with more detailed operational data and to expand their application to other types of industrial equipment.
References
Ahmad, R., & Kamaruddin, S. (2012). An overview of time-based and condition-based maintenance in industrial application. Computers & Industrial Engineering, 63(1), 135-149. https://doi.org/10.1016/j.cie.2012.02.002
Grall, A., Dieulle, L., Bérenguer, C., & Roussignol, M. (2002). Continuous-time predictive-maintenance scheduling for a deteriorating system. IEEE Transactions on Reliability, 51(2), 141-150. https://doi.org/10.1109/TR.2002.1011517
Jardine, A. K. S., Lin, D., & Banjevic, D. (2006). A review on machinery diagnostics and prognostics implementing condition-based maintenance. Mechanical Systems and Signal Processing, 20(7), 1483-1510. https://doi.org/10.1016/j.ymssp.2005.09.012
Lee, J., Lapira, E., Bagheri, B., & Kao, H. (2020). Recent advances and trends in predictive manufacturing systems in big data environment. Manufacturing Letters, 1(1), 38-41. https://doi.org/10.1016/j.mfglet.2013.09.005
Mobley, R. K. (2002). An introduction to predictive maintenance. Elsevier.