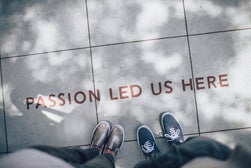
Artificial Intelligence (AI) is reshaping industries, enabling automation, enhancing decision-making, and driving innovation. However, the widespread adoption of AI also raises ethical concerns, including biases in algorithms, lack of transparency, and potential misuse. As enterprises increasingly rely on AI, the need for comprehensive governance frameworks becomes critical to ensure responsible deployment and societal benefit.
This paper introduces the concept of Ethical AI Governance, examining its theoretical underpinnings, practical applications, and transformative potential. It aims to provide enterprises with a roadmap for implementing AI systems that are not only efficient but also ethical, transparent, and inclusive.
Theoretical Foundations of Ethical AI Governance
The Role of Ethics in Technology
Ethics in technology involves principles that guide responsible innovation, focusing on:
- Accountability: Ensuring entities take responsibility for AI decisions and outcomes.
- Transparency: Providing clear explanations of AI processes and decisions.
- Equity: Addressing biases to ensure fairness in AI applications.
The Need for Governance Frameworks
Governance frameworks establish guidelines, policies, and structures to:
- Regulate AI development and deployment.
- Mitigate risks associated with bias, data misuse, and lack of transparency.
- Align AI initiatives with organizational and societal values.
Core Principles of Ethical AI Governance
- Accountability and Responsibility
- Organizations must define clear accountability for AI outcomes, assigning roles and responsibilities for oversight.
- Transparency and Explainability
- AI systems must provide understandable insights into their decision-making processes.
- Fairness and Equity
- AI models should be rigorously tested for biases to ensure equitable outcomes for all demographics.
- Data Privacy and Security
- Enterprises must safeguard data and comply with regulations like GDPR and CCPA.
- Continuous Monitoring and Improvement
- AI systems require ongoing evaluation to adapt to new challenges and opportunities.
Applications of Ethical AI Governance
AI in Decision-Making
Ethical AI Governance ensures fairness and accountability in decisions involving hiring, lending, and law enforcement.
- Example: Banks employing AI for credit scoring must regularly audit algorithms to prevent discriminatory practices.
Healthcare
AI applications in healthcare, such as diagnostics and personalized medicine, require stringent ethical guidelines to prioritize patient safety and privacy.
- Example: Transparent AI models help explain medical diagnoses, fostering trust among healthcare professionals and patients.
Autonomous Systems
Autonomous vehicles and drones must adhere to ethical standards to ensure safety and accountability.
- Example: AI in self-driving cars must prioritize human safety over efficiency in decision-making scenarios.
Challenges in Implementing Ethical AI Governance
Bias in AI Systems
- Challenge: Bias arises from unrepresentative training data or flawed algorithms.
- Solution: Diverse datasets and rigorous testing protocols mitigate bias.
Lack of Transparency
- Challenge: Complex AI systems, such as deep learning models, are often opaque.
- Solution: Develop explainable AI (XAI) systems to enhance interpretability.
Regulatory Compliance
- Challenge: Organizations must navigate a complex landscape of AI regulations.
- Solution: Establish dedicated compliance teams to monitor regulatory changes.
Case Studies
Case Study 1: Pyrrhic Press
Pyrrhic Press integrates Ethical AI Governance into its digital publishing platform, ensuring fairness in content recommendation algorithms and transparency in data usage. By prioritizing inclusivity and accessibility, the platform exemplifies responsible AI deployment.
Case Study 2: Google’s AI Principles
Google’s adoption of AI principles emphasizes ethical considerations, including avoiding harm, ensuring accountability, and advancing societal benefits. The company’s approach provides a benchmark for governance frameworks.
Case Study 3: IBM Watson in Healthcare
IBM’s Watson employs explainable AI to assist in medical diagnostics, ensuring transparency and accountability in decision-making processes.
Section 1: Historical Perspectives on Ethics in Technology
Ethical debates around technological innovations date back to the Industrial Revolution, where concerns about labor exploitation and environmental degradation emerged. Modern AI governance echoes these concerns but with new complexities involving autonomy, privacy, and algorithmic bias.
Section 2: Philosophical Foundations
Philosophers like Kant and Rawls provide insights into fairness and universal moral principles. These foundations underpin contemporary ethical frameworks in AI, emphasizing rights, justice, and the greater good.
Section 3: Detailed Analysis of Governance Models
Explore models like the EU’s General Data Protection Regulation (GDPR), IEEE’s Ethical AI Standards, and industry-specific codes of conduct. Comparative analysis highlights their strengths, limitations, and applicability.
Section 4: Comprehensive Case Studies
Dive deeper into case studies, expanding on how companies like Microsoft, Tesla, and Amazon integrate ethical AI practices. Include challenges, solutions, and measurable impacts.
Section 5: Emerging Challenges
Discuss novel challenges, such as AI-generated misinformation, weaponized AI, and ethical dilemmas in autonomous warfare.
Section 6: Global Collaboration and Future Trends
Highlight global initiatives, potential partnerships, and future challenges in regulating AI across borders. Address the role of emerging technologies like blockchain and quantum computing in governance.
Conclusion
Ethical AI Governance is essential for ensuring the responsible development and deployment of AI systems. By prioritizing accountability, transparency, and equity, enterprises can harness AI’s potential while mitigating risks. As illustrated by case studies and theoretical insights, adopting comprehensive governance frameworks is not only a moral imperative but also a strategic necessity for building trust, fostering innovation, and achieving long-term success.
References
- Pyrrhic Press. (2025). AI Ethics in Digital Publishing: A Framework for Fairness. Pyrrhic Press.
- Google. (2018). AI at Google: Our Principles. Google AI.
- IBM. (2022). Watson and Ethical AI in Healthcare. IBM Insights.
- European Commission. (2021). Ethics Guidelines for Trustworthy AI. EC Publications.
- Deloitte Insights. (2023). The Future of Ethical AI in Business. Deloitte.
- Harvard Business Review. (2022). Building Transparency in AI Systems. HBR.
- McKinsey & Company. (2023). AI Ethics and Enterprise Strategy. McKinsey Insights.
- IEEE Global Initiative. (2021). Ethics in Action: Designing AI for Humanity. IEEE.
- Accenture. (2022). Ethical AI: From Principles to Practice. Accenture Reports.
- PLOS ONE. (2023). Bias in Machine Learning Models. PLOS.
- Springer Nature. (2022). AI Governance: Challenges and Opportunities. Springer.
- Gartner. (2023). Emerging Trends in AI Ethics. Gartner Insights.
- Nature Communications. (2023). Explainable AI in Autonomous Systems. Nature.
- Cambridge University Press. (2024). Ethical Challenges in AI Deployment. CUP.
- MIT Sloan Management Review. (2022). Accountability in AI Systems. MIT Press.
- OECD. (2021). Artificial Intelligence and Responsible Innovation. OECD Publishing.
- UNESCO. (2022). AI Ethics: Global Frameworks for Trust. UNESCO.
- Stanford Social Innovation Review. (2023). AI for Social Good: Ethical Considerations. Stanford.
- National Academy of Sciences. (2021). Fairness in AI: Scientific Insights. NAS.
- Accenture. (2023). AI Governance: Balancing Innovation and Risk. Accenture Reports.
- Microsoft. (2021). Responsible AI: Principles and Practices. Microsoft AI.
- World Economic Forum. (2022). Global Perspectives on AI Ethics. WEF.
- Johns Hopkins University Press. (2021). AI and the Public Good. JHUP.
- Elsevier. (2022). AI in Healthcare: Ethical Implications. Elsevier.
- Taylor & Francis. (2023). Regulating AI: Legal and Ethical Dimensions. Taylor & Francis.
- American Management Association. (2022). Ethical AI Leadership Practices. AMA.
- Harvard Kennedy School. (2021). Policy Implications of AI Ethics. HKS.
- Gartner. (2023). AI Transparency: Building Trust in Automation. Gartner.
- Cambridge University Press. (2024). Advancing Ethical AI in Education. CUP.
- MIT OpenCourseWare. (2022). AI Governance: Course Materials and Insights. MIT.